A Machine Learning-Based algorithm for the assessment of clinical metabolomic fingerprints in Zika virus disease
Abstract:
Data analysis for metabolomic studies is challenging considering the number of statistical tools and standardization processes, which provides different results and projection in a single study. In addition, generation of high complexity data is common for untargeted metabolomics, requiring careful analysis and interpretation of results. In order to propose an innovative method for the analysis of a mass spectrometry metabolomics dataset, data from a Zika virus study was used. The analysis of this dataset combined principal component analysis and supervised learning methods such as support vector machines and logistic regression, to provide a truthful pbkp_rediction model for discriminating samples of individuals with Zika virus infection and healthy controls. These supervised methods were used to learn the features that configured the 'fingerprint' for the viral infection, showing over 98% of accuracy in a validation set. This model could be used as a fast and reliable test for determining Zika virus infections as part of healthcare services. Furthermore, this novel method shows potential for diagnosing other arboviral diseases.
Año de publicación:
2019
Keywords:
- SVM
- PCa
- logistic regression
- Zika Virus
- Machine learning
Fuente:
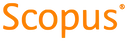
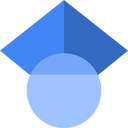
Tipo de documento:
Conference Object
Estado:
Acceso restringido
Áreas de conocimiento:
- Metabolismo
- Aprendizaje automático
- Metabolismo
Áreas temáticas:
- Fisiología humana
- Medicina y salud
- Enfermedades