Monthly tuna catches forecasting using multiscale additive autoregression
Abstract:
In this paper, a forecasting strategy based on an additive autoregressive model combined with multiscale wavelet analysis to improve the accuracy of monthly tuna catches in equatorial Indian Ocean is proposed. The general idea of the proposed forecasting model is to decompose the raw tune data set into trend and residual components by using stationary wavelet transform. In wavelet domain, the trend component and residual component are forecasted with a linear autoregressive model and a nonlinear additive autoregressive model; respectively. Hence, the proposed forecast is the co-addition of two pbkp_redicted components. We find that the proposed forecasting strategy achieves 98% of the explained variance with reduced parsimony and high accuracy. ©2009 IEEE.
Año de publicación:
2009
Keywords:
- Wavelet abalysis
- regression
- forecasting
Fuente:
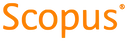
Tipo de documento:
Conference Object
Estado:
Acceso restringido
Áreas de conocimiento:
- Estadísticas
- Pronóstico
Áreas temáticas:
- Ciencias de la computación