Multi-category classification of mammograms by using convolutional neural networks
Abstract:
This work describes the process of multi-category classification of mammograms by using Convolutional Neural Networks (CNN), starting from the acquisition and preparation of the dataset, then the architecture and training of the Inception-v3 model from Google, until obtaining and analyzing our results. We employed a total of 1000 mammograms of the Digital Database for Screening Mammography (DDSM) supported by the University of South Florida, divided in 5 classes according to BI-RADS categories. The optimization of our results is through a grid search method by combining the values of the following parameters: Batch size, learning rate, optimizer, loss function and number of layers to be frozen in the Inception-v3 model. The validation metrics of our algorithm performance, given by the parameter combinations, are based mainly on a loss function and accuracy. The best performance result generates an accuracy of 97% and a loss function value of 0.02, respectively.
Año de publicación:
2018
Keywords:
- Breast Cancer
- pattern recognition
- TensorFlow
- Convolutional neural network
- BIRADS
Fuente:
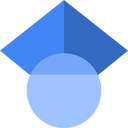
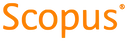
Tipo de documento:
Conference Object
Estado:
Acceso restringido
Áreas de conocimiento:
- Aprendizaje automático
- Laboratorio médico
Áreas temáticas:
- Fisiología humana
- Ciencias de la computación
- Enfermedades