Multi-label learning by hyperparameters calibration for treating class imbalance
Abstract:
Multi-label learning has been becoming an increasingly active area into the machine learning community due to a wide variety of real world problems. However, only over the past few years class balancing for these kind of problems became a topic of interest. In this paper, we present a novel method named hyperparameter calibration to treat class imbalance in a multi-label problem, to this aim we develop an extensive analysis over four real-world databases and two own synthetic databases exhibiting different ratios of imbalance. The empirical analysis shows that the proposed method is able to improve the classification performance when it is combined with three of the most widely used strategies for treating multi-label classification problems.
Año de publicación:
2018
Keywords:
- Imbalanced learning
- Multilabel classification
- Support Vector Machine
- Problem transformation
Fuente:
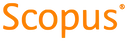
Tipo de documento:
Conference Object
Estado:
Acceso restringido
Áreas de conocimiento:
- Aprendizaje automático
Áreas temáticas:
- Programación informática, programas, datos, seguridad
- Funcionamiento de bibliotecas y archivos
- Escuelas y sus actividades; educación especial