Multi-labeler classification using kernel representations and mixture of classifiers
Abstract:
This work introduces a multi-labeler kernel novel approach for data classification learning from multiple labelers. The learning process is done by training support-vector machine classifiers using the set of labelers (one labeler per classifier). The objective functions representing the boundary decision of each classifier are mixed by means of a linear combination. Followed from a variable relevance, the weighting factors are calculated regarding kernel matrices representing each labeler. To do so, a so-called supervised kernel function is also introduced, which is used to construct kernel matrices. Our multi-labeler method reaches very good results being a suitable alternative to conventional approaches.
Año de publicación:
2017
Keywords:
- Supervised kernel
- SUPPORT VECTOR MACHINES
- Multi-labeler classification
Fuente:
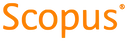
Tipo de documento:
Conference Object
Estado:
Acceso restringido
Áreas de conocimiento:
- Aprendizaje automático
Áreas temáticas:
- Ciencias de la computación