Multi-pbkp_redictor mapping of soil organic carbon in the alpine tundra: a case study for the central Ecuadorian páramo
Abstract:
Background: Soil organic carbon (SOC) affects essential biological, biochemical, and physical soil functions such as nutrient cycling, water retention, water distribution, and soil structure stability. The Andean páramo known as such a high carbon and water storage capacity ecosystem is a complex, heterogeneous and remote ecosystem complicating field studies to collect SOC data. Here, we propose a multi-pbkp_redictor remote quantification of SOC using Random Forest Regression to map SOC stock in the herbaceous páramo of the Chimborazo province, Ecuador. Results: Spectral indices derived from the Landsat-8 (L8) sensors, OLI and TIRS, topographic, geological, soil taxonomy and climate variables were used in combination with 500 in situ SOC sampling data for training and calibrating a suitable pbkp_redictive SOC model. The final pbkp_redictive model selected uses nine pbkp_redictors with a RMSE of 1.72% and a R2 of 0.82 for SOC expressed in weight %, a RMSE of 25.8 Mg/ha and a R2 of 0.77 for the model in units of Mg/ha. Satellite-derived indices such as VARIG, SLP, NDVI, NDWI, SAVI, EVI2, WDRVI, NDSI, NDMI, NBR and NBR2 were not found to be strong SOC pbkp_redictors. Relevant pbkp_redictors instead were in order of importance: geological unit, soil taxonomy, precipitation, elevation, orientation, slope length and steepness (LS Factor), Bare Soil Index (BI), average annual temperature and TOA Brightness Temperature. Conclusions: Variables such as the BI index derived from satellite images and the LS factor from the DEM increase the SOC mapping accuracy. The mapping results show that over 57% of the study area contains high concentrations of SOC, between 150 and 205 Mg/ha, positioning the herbaceous páramo as an ecosystem of global importance. The results obtained with this study can be used to extent the SOC mapping in the whole herbaceous ecosystem of Ecuador offering an efficient and accurate methodology without the need for intensive in situ sampling.
Año de publicación:
2021
Keywords:
- Landsat
- Carbon stock mapping
- vegetation indices
- Random forest regression
- Soil organic carbon (SOC)
- Multispectral indices
Fuente:
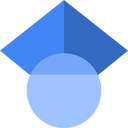
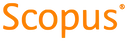
Tipo de documento:
Article
Estado:
Acceso abierto
Áreas de conocimiento:
- Mecánica de suelos
- Fertilidad del suelo
- Ecología
Áreas temáticas:
- Técnicas, equipos y materiales