Multi-resolution SVD, Linear Regression, and Extreme Learning Machine for Traffic Accidents Forecasting with Climatic Variable
Abstract:
The purpose of this work is to forecast variables related to traffic accidents in Ecuador since 2015 to 2019. Traffic accidents lead severe injuries and fatalities in Ecuador with 4925 deaths and 20794 injured in the period of analysis. Models based on Multiresolution Singular Value Decomposition (MSVD) and Extreme Learning Machine (ELM) are proposed to improve the accuracy for multi-week ahead forecasting. This study adds a climatic variable for enhancing the effectiveness of both type of models. The performance of MSVD+ELM based is compared with a conventional Linear Regression Model (LRM) joint with MSVD. To assess the forecasting accuracy, three metrics were used, Root Mean Squared Error (RMSE), Index of Agreement modified (IoAm), and Nash-Suctlife Efficiency modified (NSEm). Models based on Linear Regression (SVD+LRM) without climatic variable present the lowest accuracy, with an average Nash-Suctlife Efficiency of 65.4% for 12-weeks ahead forecasting, whereas models that integrate climatic variable at input, present gains in pbkp_rediction accuracies, with an average Nash-Suctlife Efficiency of 94.6% for Linear Regression - based models, and 95.9%. for ELM -based models. The implementation of the proposed models will help to guide the planning of government institutions and decision-making, in face of complex problem of traffic accidents addressed in this work.
Año de publicación:
2022
Keywords:
- Multiresolution singular value decomposition
- Extreme learning machine
- linear regression
- forecasting
- traffic accidents
Fuente:
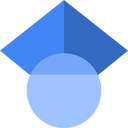
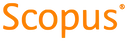
Tipo de documento:
Conference Object
Estado:
Acceso restringido
Áreas de conocimiento:
- Ciencias de la computación
- Aprendizaje automático
- Clima
Áreas temáticas:
- Ciencias de la computación