Multi-source and multimodal data fusion for predicting academic performance in blended learning university courses
Abstract:
In this paper we apply data fusion approaches for predicting the final academic performance of university students using multiple-source, multimodal data from blended learning environments. We collect and preprocess data about first-year university students from different sources: theory classes, practical sessions, on-line Moodle sessions, and a final exam. Our objective is to discover which data fusion approach produces the best results using our data. We carry out experiments by applying four different data fusion approaches and six classification algorithms. The results show that the best predictions are produced using ensembles and selecting the best attributes approach with discretized data. The best prediction models show us that the level of attention in theory classes, scores in Moodle quizzes, and the level of activity in Moodle forums are the best set of attributes for predicting students’ final performance in our courses.
Año de publicación:
2021
Keywords:
- Pbkp_redicting academic performance
- multimodal learning
- Multisource data
- data fusion
- BLENDED LEARNING
Fuente:
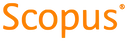
Tipo de documento:
Article
Estado:
Acceso restringido
Áreas de conocimiento:
- Tecnología educativa
Áreas temáticas de Dewey:
- Ciencias de la computación
- Escuelas y sus actividades; educación especial
- Educación superior

Objetivos de Desarrollo Sostenible:
- ODS 4: Educación de calidad
- ODS 17: Alianzas para lograr los objetivos
- ODS 9: Industria, innovación e infraestructura
