Multivariate analysis and characterization of low impact crime in Mexico City
Abstract:
Background: Multivariate analysis is fast becoming a key instrument that can be used to address crimes or incidents. It may be helpful to assess government policies on crime prevention. Methods: To distinguish between the 25 official crime categories reported in Mexico City (Jan 2019 - Jun 2019), principal coordinates analysis was used to determine the quality of a characteristic in this context. This study used cluster analysis via K-means and Biplot based on time and location in terms of crime occurrence. Results: The results obtained from this preliminary analysis indicates that around 70% of crime occurrence is shown in the following boroughs: Cuauhtémoc, Iztapalapa, Gustavo A. Madero, Benito Juárez, Álvaro Obregón, Coyoacán, and Miguel Hidalgo. Conclusion: There are two factors that contribute to the difficulties in crime analysis in Mexico City, namely, the lack of people’s trust in authorities and the insufficiency of tools for data analysis. The latter is an integral part in achieving justice for the victims of crimes because it impedes the process of observing patterns and pbkp_redicting the perpetrators’ next actions, which may help in solving a number of types of crimes. It is then imperative for law enforcement to utilize data analysis tools that aid in identifying crime patterns and trends, such that the occurrences of crime show a downward trend and consequentially increase the people’s trust in law enforcement agencies.
Año de publicación:
2021
Keywords:
- biplot
- k-means clustering
- crime analysis
- Principal coordinates analysis
- Multivariate analysis
Fuente:
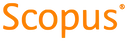
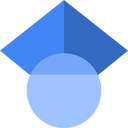
Tipo de documento:
Article
Estado:
Acceso abierto
Áreas de conocimiento:
- Crimen
Áreas temáticas:
- Otros problemas y servicios sociales