Multivariate regression with incremental learning of Gaussian mixture models
Abstract:
Within the machine learning framework, incremental learning of multivariate spaces is of special interest for on-line applications. In this work, the regression problem for multivariate systems is solved by implementing an efficient probabilistic incremental algorithm. It allows learning high-dimensional redundant non-linear maps by the cumulative acquisition of data from input-output systems. The proposed model is aimed at solving pbkp_rediction and inference problems. The implementation introduced in this work allows learning from data batches without the need of keeping them in memory afterwards. The learning architecture is built using Incremental Gaussian Mixture Models. The Expectation-Maximization algorithm and general geometric properties of Gaussian distributions are used to train the models. Our current implementation can produce accurate results fitting models in real multivariate systems. Results are shown from testing the algorithm for both situations, one where the incremental learning is demonstrated and the second where the performance to solve the regression problem is evaluated on a toy example.
Año de publicación:
2017
Keywords:
- Regresssion problem
- Incremental learning
- Gaussian Mixture Models
Fuente:
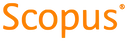
Tipo de documento:
Conference Object
Estado:
Acceso restringido
Áreas de conocimiento:
- Aprendizaje automático
- Optimización matemática
- Estadísticas
Áreas temáticas:
- Programación informática, programas, datos, seguridad