NISS
Abstract:
There is clear value, in both industrial and government settings, derived from performing statistical analyses that, in effect, integrate data in multiple, distributed databases. However, the barriers to actually integrating the data can be substantial or even insurmountable. Corporations may be unwilling to share proprietary databases such as chemical databases held by pharmaceutical manufacturers, government agencies are subject to laws protecting confidentiality of data subjects, and even the sheer volume of the data may preclude actual data integration. In this paper, we show how tools from modern information technology—specifically, secure multiparty computation and networking—can be used to perform statistically valid analyses of distributed databases. The common characteristic of the methods we describe is that the owners share sufficient statistics computed on the local databases in a way that protects each owner from the others. That is, while each owner can calculate the “complement” of its contribution to the analysis, it cannot discern which other owners contributed what to that complement. Our focus is on horizontally partitioned data: the data records rather than the data attributes are spread among the owners. We present protocols for secure regression, contingency tables, maximum likelihood and Bayesian analysis. For low-risk situations, we describe a secure data integration protocol that integrates the databases but prevents owners from learning the source of data records other than their own. Finally, we outline three current research directions: a software system implementing the protocols, secure EM algorithms, and partially …
Año de publicación:
2005
Keywords:
Fuente:
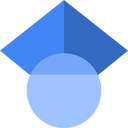
Tipo de documento:
Other
Estado:
Acceso abierto
Áreas de conocimiento:
Áreas temáticas de Dewey:

Objetivos de Desarrollo Sostenible:
- ODS 9: Industria, innovación e infraestructura
- ODS 17: Alianzas para lograr los objetivos
- ODS 8: Trabajo decente y crecimiento económico
