Natural Rubber Blend Optimization via Data-Driven Modeling: The Implementation for Reverse Engineering
Abstract:
Natural rubber formulation methodologies implemented within industry primarily implicate a high dependence on the formulator’s experience as it involves an educated guess-and-check process. The formulator must leverage their experience to ensure that the number of iterations to the final blend composition is minimized. The study presented in this paper includes the implementation of blend formulation methodology that targets material properties relevant to the application in which the product will be used by incorporating pbkp_redictive models, including linear regression, response surface method (RSM), artificial neural networks (ANNs), and Gaussian process regression (GPR). Training of such models requires data, which is equal to financial resources in industry. To ensure minimum experimental effort, the dataset is kept small, and the model complexity is kept simple, and as a proof of concept, the pbkp_redictive models are used to reverse engineer a current material used in the footwear industry based on target viscoelastic properties (relaxation behavior, tan δ, and hardness), which all depend on the amount of crosslinker, plasticizer, and the quantity of voids used to create the lightweight high-performance material. RSM, ANN, and GPR result in pbkp_rediction accuracy of 90%, 97%, and 100%, respectively. It is evident that the testing accuracy increases with algorithm complexity; therefore, these methodologies provide a wide range of tools capable of pbkp_redicting compound formulation based on specified target properties, and with a wide range of complexity.
Año de publicación:
2022
Keywords:
- formulation
- Reverse Engineering
- Machine learning
- Viscoelasticity
- Optimization
- Natural rubber
- Modeling
- Response Surface Methodology
Fuente:
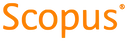
Tipo de documento:
Article
Estado:
Acceso abierto
Áreas de conocimiento:
- Ciencia de materiales
- Optimización matemática
Áreas temáticas:
- Métodos informáticos especiales