Near-real-time satellite precipitation data ingestion into peak runoff forecasting models
Abstract:
Extreme peak runoff forecasting is still a challenge in hydrology. In fact, the use of traditional physically-based models is limited by the lack of sufficient data and the complexity of the inner hydrological processes. Here, we employ a Machine Learning technique, the Random Forest (RF) together with a combination of Feature Engineering (FE) strategies for adding physical knowledge to RF models and improving their forecasting performances. The FE strategies include precipitation-event classification according to hydrometeorological criteria and separation of flows into baseflow and directflow. We used ∼ 3.5 years of hourly precipitation information retrieved from two near-real-time satellite precipitation databases (PERSIANN-CCS and IMERG-ER), and runoff data at the outlet of a 3391-km2 basin located in the tropical Andes of Ecuador. The developed models obtained Nash-Sutcliffe efficiencies varying from 0.86 to 0.59 for lead times between 1 and 6 h. The best performances were obtained for peak runoffs triggered by short-extension precipitation events (<50 km2) where infiltration- or saturation-excess runoff responses are well learned by the RF models. Conversely, the forecasting difficulty is associated with extensive precipitation events. For such conditions, a deeper characterization of the biophysical characteristics of the basin is encouraged for capturing the dynamic of directflow across multiple runoff responses. All in all, the potential to employ near-real-time satellite precipitation and the use of FE strategies for improving RF forecasting provides hydrologists with new tools for real-time runoff forecasting in remote or complex regions.
Año de publicación:
2023
Keywords:
- forecasting
- Extreme runoff
- Baseflow separation
- PERSIANN
- IMERG
- Feature engineering
- Tropical Andes
Fuente:
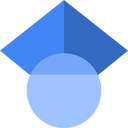
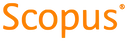
Tipo de documento:
Article
Estado:
Acceso restringido
Áreas de conocimiento:
- Hidráulica
- Sensores remotos
- Sensores remotos
Áreas temáticas:
- Ciencias de la computación