Neighborhood criterion analysis for prototype selection applied in WSN data
Abstract:
The present work presents an analysis of the neighborhood criterion for the prototype selection (PS) in supervised machine learning classification algorithms. To do this, we use the condensed neighbor algorithm CNN to eliminate redundant data with the normalization of the distance to the centroid of each data subset criterion. This is done, in order to obtain the training matrix of the most optimal model. A selection of neighborhood criterion has been created from the quantification of the balance between the performance of the classification and the reduction of data set (CER). As proof of the test, we performed: (i) CER and (ii) real-time tests with the implementation of the algorithm within the WSN. The result is a data reduction of up to 88 % and a performance of the kNN classifier of 75%. It is concluded that the criterion of neighborhood with normalized distance must be less than or equal to 0.2 and the implementation of kNN with k = 1 obtains the best CER.
Año de publicación:
2018
Keywords:
- prototype selection
- Knn
- cnn
- nn
Fuente:
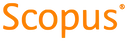
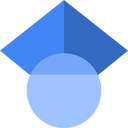
Tipo de documento:
Conference Object
Estado:
Acceso restringido
Áreas de conocimiento:
- Minería de datos
- Ciencias de la computación
Áreas temáticas de Dewey:
- Sistemas