Neural network feature and architecture optimization for injection molding surface defect prediction of model polypropylene
Abstract:
The industry is heading towards digitalization with production and quality assurance of processes and products. Novel practices such as machine learning or artificial intelligence make it possible for highly complex and nonlinear occurrences to be modeled and predicted with immense accuracy when real experiences are used to train the algorithm. For example, supervised learning in a closed-loop system allows the user to analyze and predict outcomes and gives it the ability to adapt and add intelligence to the current system. This study focuses on the development of a neural network (NN) for surface defect prediction in injection molding of model polypropylene. Feature optimization allows us to conclude that rheological parameters such as the melt flow index and relaxation time ((Formula presented.)) can improve predictive accuracy. Furthermore, Bayesian optimization is implemented to optimize the NN structure. The optimization approach allowed for a cross-validation (CV) accuracy of 90.2% ± 4.4% with only five input parameters, while the seven-input parameter optimized structure arrived at a CV accuracy of 92.4% ± 11.4%. Although the full-feature structure optimized with Bayesian optimization concluded with slightly higher accuracy, the error range dramatically increased, meaning that this structure tends to overfit.
Año de publicación:
2021
Keywords:
- classification
- injection molding
- rheology
- MORPHOLOGY
- viscoelastic properties
Fuente:
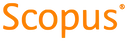
Tipo de documento:
Article
Estado:
Acceso abierto
Áreas de conocimiento:
- Ingeniería de manufactura
- Aprendizaje automático
Áreas temáticas de Dewey:
- Métodos informáticos especiales
- Física aplicada
- Instrumentos de precisión y otros dispositivos

Objetivos de Desarrollo Sostenible:
- ODS 9: Industria, innovación e infraestructura
- ODS 12: Producción y consumo responsables
- ODS 8: Trabajo decente y crecimiento económico
