Neural semi-supervised learning for multi-labeled short-texts
Abstract:
The massive data generated by users in online platforms, such as social networks, create challenges for text classification tasks based on supervised learning. Supervised learning often requires a lot of feature engineering or a significant amount of annotated data to achieve good results. However, the scarcity of annotated data is a critical issue, and manual annotation can be both costly and time-consuming. Semi-supervised learning requires far less annotated data and achieve similar performance as supervised approaches. In this paper, we introduce a semi-supervised neural architecture for muti-label settings, that combines deep learning representation and k-means clustering. The results show that the semi-supervised approach can leverage large-scale unlabeled data and achieve better results compared to baseline unsupervised as well as supervised methods.
Año de publicación:
2019
Keywords:
Fuente:
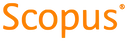
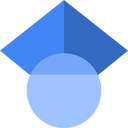
Tipo de documento:
Conference Object
Estado:
Acceso restringido
Áreas de conocimiento:
- Aprendizaje automático
- Ciencias de la computación
Áreas temáticas de Dewey:
- Programación informática, programas, datos, seguridad
- Métodos informáticos especiales
- Funcionamiento de bibliotecas y archivos

Objetivos de Desarrollo Sostenible:
- ODS 9: Industria, innovación e infraestructura
- ODS 4: Educación de calidad
- ODS 8: Trabajo decente y crecimiento económico
