Neural-network security-boundary constrained optimal power flow
Abstract:
This paper proposes a new approach to model stability and security constraints in optimal power flow (OPF) problems based on a neural network (NN) representation of the system security boundary (SB). The novelty of this proposal is that a closed form, differentiable function derived from the system's SB is used to represent security constraints in an OPF model. The procedure involves two main steps: First, an NN representation of the SB is obtained based on back-propagation neural network (BPNN) training. Second, a differentiable mapping function extracted from the BPNN is used to directly incorporate this function as a constraint in the OPF model. This approach ensures that the operating points resulting from the OPF solution process are within a feasible and secure region, whose limits are better represented using the proposed technique compared to typical security-constrained OPF models. The effectiveness and feasibility of the proposed approach is demonstrated through the implementation, as well as testing and comparison using the IEEE two-area and 118-bus benchmark systems, of an optimal dispatch technique that guarantees system security in the context of competitive electricity markets. © 2010 IEEE.
Año de publicación:
2011
Keywords:
- Power System Stability
- optimal power flow
- Power system security
- neural network
Fuente:
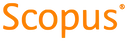
Tipo de documento:
Article
Estado:
Acceso restringido
Áreas de conocimiento:
- Inteligencia artificial
- Ingeniería energética
Áreas temáticas:
- Métodos informáticos especiales
- Ciencias de la computación
- Física aplicada