A Multi-object Deep Neural Network Architecture to detect Prostate Anatomy in T2-weighted MRI: Performance Evaluation
Abstract:
We present a 2D-3D convolutional neural network (CNN) ensemble automatically constructed to segment the whole prostate gland along with the peripheral zone (PZ) (PPZ-SegNet) using T2-weighted sequence of MRI. The study used four different data sets all obtained from The Cancer Imaging Archive (TCIA). The prostate gland and the zonal anatomy (PZ) were manually delineated by consensus read through a clinical reader, using the axial T2W for three data sets. In this work, the PPZ-SegNet was trained using 150 cases of ProstateX data with prostate and PZ annotations. The model evaluation was performed on an independent cohort of 283 T2W MRI prostate cases, obtained from a diverse cohort. The segmentation performance was evaluated by computing the dice similarity coefficient and Hausdorff distance between the estimated-deep-network identified regions and the radiologist drawn annotations. We …
Año de publicación:
Keywords:
Fuente:
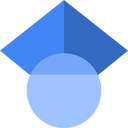
Tipo de documento:
Other
Estado:
Acceso abierto
Áreas de conocimiento:
- Ciencias de la computación
Áreas temáticas:
- Fisiología humana