Non-linear global optimization via parameterization and inverse function approximation: An artificial neural networks approach
Abstract:
In this article, a novel technique for non-linear global optimization is presented. The main goal is to find the optimal global solution of non-linear problems avoiding sub-optimal local solutions or inflection points. The proposed technique is based on a two steps concept: properly keep decreasing the value of the objective function, and calculating the corresponding independent variables by approximating its inverse function. The decreasing process can continue even after reaching local minima and, in general, the algorithm stops when converging to solutions near the global minimum. The implementation of the proposed technique by conventional numerical methods may require a considerable computational effort on the approximation of the inverse function. Thus, here a novel Artificial Neural Network (ANN) approach is implemented to reduce the computational requirements of the proposed optimization technique. This approach is successfully tested on some highly non-linear functions possessing several local minima. The results obtained demonstrate that the proposed approach compares favorably over some current conventional numerical (Matlab functions) methods, and other non-conventional (Evolutionary Algorithms, Simulated Annealing) optimization methods. © World Scientific Publishing Company.
Año de publicación:
2007
Keywords:
- Global optimization
- Inverse functions
- artificial neural networks
- non-linear programming
Fuente:
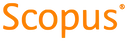
Tipo de documento:
Article
Estado:
Acceso restringido
Áreas de conocimiento:
- Algoritmo
- Red neuronal artificial
- Algoritmo
Áreas temáticas:
- Programación informática, programas, datos, seguridad