Nonintrusive, self-organizing, and probabilistic classification and identification of plugged-in electric loads
Abstract:
Electricity consumption for plugged-in electric loads (PELs) accounts for more use than any other single end-use service in residential and commercial buildings. PELs possess potentials to be efficiently managed for many purposes. However, few existing load identification methods are designed for PELs to handle challenges such as the diversity within each type of PELs and similarity between different types of PELs with similar front-end power supply units. Existing methods provide only absolute decisions which are not reliable when handling these challenges. This paper presents a simple yet efficient and practical hybrid supervised self-organizing map (SSOM)/Bayesian identifier for PELs. The proposed identifier can classify PELs into clusters by inherent similarities due to similar front-end power supply topologies, extract and utilize statistical information, and provide the probability of the unknown load belonging to a specific type of load. Tests based on real-world data validate that the proposed methods are accurate, robust, and applicable. © 2010-2012 IEEE.
Año de publicación:
2013
Keywords:
- high efficiency buildings
- nonintrusive load monitoring
- self-organizing map
- Electric load identification
Fuente:
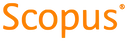
Tipo de documento:
Article
Estado:
Acceso restringido
Áreas de conocimiento:
- Potencia eléctrica
- Aprendizaje automático
- Simulación por computadora
Áreas temáticas:
- Programación informática, programas, datos, seguridad
- Física aplicada
- Probabilidades y matemática aplicada