Nonlinear autoregressive with exogenous inputsneural network for water level pbkp_rediction
Abstract:
Water level pbkp_rediction is a flood disaster issues which needs to have sufficient time for low-land communities to prepare and secure their lives and properties. This study aims to pbkp_redict flood water level for longer term forecasting using Artificial Neural Network (ANN). Specifically, it aims todetermine exogenous inputs that contribute to the pbkp_rediction of flood water level; determine additional set of criterion in the selection of optimized ANN model and determine the best fit mathematical model for each optimized ANN model. The ANN models were trained using NNtool of Matlab with the default Levenberg-Marquardt as training algorithm. Eleven cases were formulated to determine the optimized model. Nonlinear autoregressive network with exogenous input (NARX) was used in modellingall cases. Selection of optimized models was based on multi-criteria approach such as: Pearson R, mean absolute error (MAE), root-mean-square error (RMSE), Akiake's Final Pbkp_rediction Error (FPE), and Nash-Sutcliffe's coefficient of efficiency (NSE). Result of the study shows that aside from upper stream, the tide level and rainfall were the exogenous inputs that contribute in pbkp_redicting the water level at flood risk areas. The optimize model for both flood risk areas is a NARX model having upper stream water as the exogenous input that has the following multi-criteria values:for medium risk flood area, the optimized model has the following criterion values: MSE=0.000153, R=0.89519, FPE= 7.1355e-7, MAE=0.0066, RMSE=0.0089 and NSE=0.7868; and for high risk flood area: MSE=1.68e-5, R=0.85153, FPE=3.5245e-7, MAE=0.0027, RMSE=0.0035, and MSE=0.5729. This study is beneficial tool to local government unitas an alternative way of pbkp_redicting and interpreting water level data to have an immediate warning to the community.
Año de publicación:
2019
Keywords:
- TIME SERIES
- NARX
- ERROR
- pbkp_rediction
- Artificial Neural Network
- water level
Fuente:
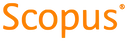
Tipo de documento:
Conference Object
Estado:
Acceso restringido
Áreas de conocimiento:
- Ciencias de la computación
- Aprendizaje automático
- Hidrología
Áreas temáticas:
- Métodos informáticos especiales