Nonlinear system identification with composite relevance vector machines
Abstract:
Nonlinear system identification based on relevance vector machines (RVMs) has been traditionally addressed by stacking the input and/or output regressors and then performing standard RVM regression. This letter introduces a full family of composite kernels in order to integrate the input and output information in the mapping function efficiently and hence generalize the standard approach. An improved trade-off between accuracy and sparsity is obtained in several benchmark problems. Also, the RVM yields confidence intervals for the predictions, and it is less sensitive to free parameter selection. © 2007 IEEE.
Año de publicación:
2007
Keywords:
- Relevance vector machine (RVM)
- Nonlinear system identification
- Composite kernels
Fuente:
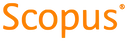
Tipo de documento:
Article
Estado:
Acceso restringido
Áreas de conocimiento:
- Aprendizaje automático
- Optimización matemática
Áreas temáticas de Dewey:
- Métodos informáticos especiales

Objetivos de Desarrollo Sostenible:
- ODS 9: Industria, innovación e infraestructura
- ODS 17: Alianzas para lograr los objetivos
- ODS 4: Educación de calidad
