Nuevo algoritmo de estimación de distribuciones con diferentes métodos de aprendizaje aplicado a planificación de proyectos
Abstract:
This paper aims at proposing a Constraint-based Learning Estimation of Distribution Algorithm (EDA) to solve the Multi-Mode Resource Constrained Project Scheduling Problem (MMRCPSP). This problem is considered a combinatorial optimization problem and consists of a set of tasks to be carried out in an ordered way, limited availability of resources, and an execution mode for each task. Generally, traditional EDAs handle the constraints of the problem using strategies such as penalty, Pareto dominance, or others, without taking into account these constraints during the learning process. These traditional approaches usually cause the generation of infeasible solutions in each iteration. In this paper, an approach based on the constraints handling during the learning process of the probabilistic model is proposed. To validate the performance of the algorithms, the new approach in two scenarios is compared. First, in the solution of a set of classical optimization problems, where different EDAs are compared in terms of efficiency and efficacy. The second group of experiments is performed on a set of instances of the PSPLIB database. In this case, the proposed algorithm is compared with a traditional EDA and with other results reported in the bibliography. The experimental results demonstrate that the proposal outperform both the results of traditional EDA and other EDA reported in the literature.
Año de publicación:
2020
Keywords:
- scheduling
- Metaheurístic
- Estimation of Distribution Algorithms
- Constraints handling
Fuente:
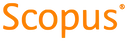
Tipo de documento:
Article
Estado:
Acceso restringido
Áreas de conocimiento:
- Algoritmo
- Algoritmo
- Investigación cuantitativa
Áreas temáticas de Dewey:
- Métodos informáticos especiales
- Programación informática, programas, datos, seguridad
- Dirección general

Objetivos de Desarrollo Sostenible:
- ODS 9: Industria, innovación e infraestructura
- ODS 17: Alianzas para lograr los objetivos
- ODS 8: Trabajo decente y crecimiento económico
