On the Use of Convolutional Neural Network Architectures for Facial Emotion Recognition
Abstract:
This work compares face gesture recognition methods based on deep learning convolutional neural network and autoencoder architectures named DCNN1, DCNN2, DCNN3, DCNN4, and DCNN+Autoencoder, that maximize the classification performance on single and mixing databases. We validated the proposed architectures on four different databases: Jaffe, CK+, FACES, and the combination of them over a five-fold cross-validation strategy. The DCNN4 was the best model in the Jaffe and FACES databases, obtaining accuracy scores of 95 % and 97 %, respectively. The DCNN2 achieved the best accuracy performance of 94 % in the CK+ database. Finally, the DCNN+Autoencoder stands as the best model in the combination of all databases (Jaffe & CK+ & FACES), achieving an accuracy score of 92 %. Moreover, according to the cross-entropy loss function, the best model per database did not incur overfitting.
Año de publicación:
2022
Keywords:
- Face gesture classification
- Face images
- Artificial Intelligence
- face emotion recognition
- deep-learning models
Fuente:
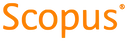
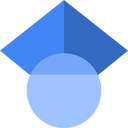
Tipo de documento:
Conference Object
Estado:
Acceso restringido
Áreas de conocimiento:
- Red neuronal artificial
- Ciencias de la computación
- Ciencias de la computación
Áreas temáticas:
- Métodos informáticos especiales
- Ciencias de la computación
- Ingeniería y operaciones afines