On the integration of molecular dynamics, data science, and experiments for studying solvent effects on catalysis
Abstract:
Computational workflows that combine molecular dynamics (MD) simulations and emerging data-centric (DC) methods can accelerate the screening and analysis of solvent systems experimentally and computationally. MD simulations provide atomic positions and velocities of reactant, solvent, and catalyst materials that can be manipulated into data representations that in turn can be used by DC techniques to conduct pbkp_redictive modeling, feature extraction, and experimental design. For liquid-phase catalytic applications, emerging DC techniques such as Convolutional and Graph Neural Networks (CNN/GNN), Topological Data Analysis (TDA), and Active Learning (AL) can leverage MD and experimental data to quickly pbkp_redict solvent effects on reaction outcomes. For instance, in recent studies, 3D solvent environments obtained with MD have been exploited by CNNs to pbkp_redict experimental reaction rates for homogeneous acid-catalyzed lignocellulosic processes. In this perspective, we discuss basic principles of DC methods and how these can be combined with MD to enable high-throughput screening of solvent selection for diverse catalysis applications.
Año de publicación:
2022
Keywords:
Fuente:
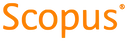
Tipo de documento:
Review
Estado:
Acceso restringido
Áreas de conocimiento:
- Catálisis
Áreas temáticas:
- Miscelánea
- Química física
- Ingeniería química