On the uncertainty modelling for linear continuous-time systems utilising sampled data and Gaussian mixture models
Abstract:
In this paper a Maximum Likelihood estimation algorithm for model error modelling in a continuous-time system is developed utilising sampled data and a Stochastic Embedding approach. Orthonormal basis functions are used to model both the continuous-time nominal model and the error-model. The stochastic properties of the error-model distribution are defined by using a Gaussian mixture model. For the estimation of the nominal model and the error-model distribution we develop a technique based on the Expectation-Maximization algorithm using sampled data from independent experiments. The benefits of our proposal are illustrated via numerical simulations.
Año de publicación:
2021
Keywords:
- Maximum likelihood
- Stochastic embedding
- Gaussian Mixture Model
- continuous-time model
- Discrete-time model
Fuente:
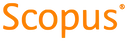
Tipo de documento:
Conference Object
Estado:
Acceso abierto
Áreas de conocimiento:
- Optimización matemática
- Optimización matemática
Áreas temáticas de Dewey:
- Ciencias de la computación

Objetivos de Desarrollo Sostenible:
