On the use of Phone-based Embeddings for Language Recognition
Abstract:
Language Identification (LID) can be defined as the process of automatically identifying the language of a given spoken utterance. We have focused in a phonotactic approach in which the system input is the phoneme sequence generated by a speech recognizer (ASR), but instead of phonemes, we have used phonetic units that contain context information, the socalled "phone-gram sequences". In this context, we propose the use of Neural Embeddings (NEs) as features for those phone-grams sequences, which are used as entries in a classical i-Vector framework to train a multi class logistic classifier. These NEs incorporate information from the neighbouring phone-grams in the sequence and model implicitly longer-context information. The NEs have been trained using both a Skip-Gram and a Glove Model. Experiments have been carried out on the KALAKA-3 database and we have used Cavg as metric to compare the systems. We propose as baseline the Cavg obtained using the NEs as features in the LID task, 24,7%. Our strategy to incorporate information from the neighbouring phone-grams to define the final sequences contributes to obtain up to 24,3% relative improvement over the baseline using Skip-Gram model and up to 32,4% using Glove model. Finally, the fusion of our best system with a MFCC-based acoustic i- Vector system provides up to 34,1% improvement over the acoustic system alone.
Año de publicación:
2018
Keywords:
- Language Identification
- neural embeddings
- Phonotactic
Fuente:
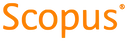
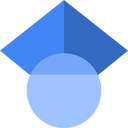
Tipo de documento:
Conference Object
Estado:
Acceso restringido
Áreas de conocimiento:
- Aprendizaje automático
- Ciencias de la computación
Áreas temáticas:
- Lenguajes de señas