On the use of multi-class support vector machines for classification of seismic signals at Cotopaxi volcano
Abstract:
This paper presents an automatic system based on machine learning algorithms for recognition of seismo-volcanic signals, such as long-period events and volcano-tectonic earthquakes, as well as signals of non-volcanic origin, like lightnings and background noise (BN). The approach is divided into two stages. A detection stage based on a decision tree algorithm, and a classification stage using Support Vector Machine in its multi-class mode. For the last, the kernel function, methods for hyperplane separability, and trade-off factor C, were evaluated. A database of seismic records collected by a seismic network deployed at Cotopaxi volcano, Ecuador, was used for testing. The approach considers the energy of the coefficients given by the wavelet transform as main features in order to distinguish events in volcanic seismograms. The detection stage was able to identify events from BN with 98% accuracy, meanwhile the classification stage reached 90% of accuracy. The optimal parameters that maximize the performance classification were the linear kernel, with a trade-off from 10 to 80, and Sequential Minimal Optimization.
Año de publicación:
2017
Keywords:
- signal detection
- wavelet transforms
- Seismic signal processing
- Pattern classification
- Real-time systems
Fuente:
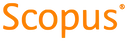
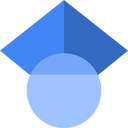
Tipo de documento:
Conference Object
Estado:
Acceso restringido
Áreas de conocimiento:
- Sismología
- Aprendizaje automático
- Sismología
Áreas temáticas:
- Geología, hidrología, meteorología
- Física aplicada
- Ciencias de la tierra