Online Dictionary Learning Based Fault and Cyber Attack Detection for Power Systems
Abstract:
The emerging wide area monitoring systems (WAMS) have brought significant improvements in electric grids' situational awareness. However, the newly introduced system can potentially increase the risk of cyber-attacks, which may be disguised as normal physical disturbances. This paper deals with the event and intrusion detection problem by leveraging a stream data mining classifier (Hoeffding adaptive tree) with semi-supervised learning techniques to distinguish cyber-attacks from regular system perturbations accurately. First, our proposed approach builds a dictionary by learning higher-level features from unlabeled data. Then, the labeled data are represented as sparse linear combinations of learned dictionary atoms. We capitalize on those sparse codes to train the online classifier along with efficient change detectors. We conduct numerical experiments with industrial control systems cyber-attack datasets. We consider five different scenarios: short-circuit faults, line maintenance, remote tripping command injection, relay setting change, as well as false data injection. The data are generated based on a modified IEEE 9-bus system. Simulation results show that our proposed approach outperforms the state-of-the-art method.
Año de publicación:
2021
Keywords:
Fuente:
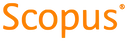
Tipo de documento:
Conference Object
Estado:
Acceso restringido
Áreas de conocimiento:
- Potencia eléctrica
- Análisis de datos
Áreas temáticas:
- Física aplicada
- Relaciones internacionales
- Ciencias de la computación