Operational photovoltaics power forecasting using seasonal time series ensemble
Abstract:
The hypothesis, namely, ensemble forecasts improve forecast accuracy, is herein investigated. More specifically, this paper considers an application of day-ahead operational PV power output forecasting using time series ensembles. Since numerical weather pbkp_rediction (NWP) is strongly favored for day-ahead solar forecasting, the motivation of using seasonal time series forecasting models is made clear at the beginning of the paper. A total of 142 models from six families are considered: the SARIMA family of models (36 models), ETS family of models (30 models), MLP (1 model), STL decomposition (2 models), TBATS family of models (72 models) and the theta model (1 model), see main text for descriptions. These models first undergo a within-group competition judged using the Akaike information criterion (AIC). The forecasts made by the six winning models, one from each family, are then combined using eight different methods: (1) simple averaging, (2) variance-based combination, (3) combination through ordinary least squares regression, (4) least absolute deviation regression, (5) constrained least squares regression, (6) complete subset regressions, (7) AIC-weighted subset regressions, and (8) lasso regression. Methods (3) to (8) cover most aspects of regression-based forecast combinations. The heavy empirical evidence from the case study suggests that the ensemble forecasting using seasonal time series models only provides marginal improvements over the best component model, in the day-ahead forecasting exercise. To that end, the pitfalls of using only time series ensembles are subsequently identified and discussed. A simple remedy, namely, adding an (uncorrected) NWP model to the ensemble, is proposed. The refined ensemble results show significant improvements over the best component model, due to the expanded information set provided by the NWP model. When this additional information set is exploited, e.g., adding a model-output-statistics-corrected NWP model, further improvements on ensemble-forecast accuracy are observed. Although a theoretical discussion on the benefits of ensemble forecasting is lacking in this paper, based on the empirical study, it appears that using forecast combination is less risky than using the best component model.
Año de publicación:
2018
Keywords:
- NWP
- TIME SERIES
- Ensemble forecast
- Forecast combination
Fuente:
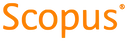
Tipo de documento:
Article
Estado:
Acceso restringido
Áreas de conocimiento:
- Fotovoltaica
- Energía
- Energía
Áreas temáticas:
- Física aplicada