Optimization of an Autonomous Learning Model for Detection COVID-19 Using Medical Images
Abstract:
Since COVID-19 appeared in 2019, detection methods based on medical images depend on the criteria of the specialist. This paper shows the optimization process of an autonomous image-based learning system. To improve the classification of positive images for COVID-19, obtained from a simple chest tomography, expert judgment and singular value decomposition are used. A computed tomography scan generates images in dicom format that must be converted to jpg or bmp format, so it is necessary to determine which format has better resolution. With the new processed images, matrix of size 145 × 145, a dataset is formed to train the neural network. When obtaining the neural network model, a reduction in training times between processed and unprocessed images is observed. Likewise, the pbkp_redictions of the neural network, developed in python, for the detection of COVID-19 with processed images indicate results over 98%. Another important result is that singular value decomposition can be used to determine that images in bmp format provide more detail than images in jpg format, working with 90% of the original information in the image. Finally, the image classification is improved and the neural network is optimized.
Año de publicación:
2022
Keywords:
- covid-19
- neural network
- Optimization
Fuente:
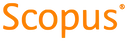
Tipo de documento:
Conference Object
Estado:
Acceso restringido
Áreas de conocimiento:
- Aprendizaje automático
- Laboratorio médico
- Ciencias de la computación
Áreas temáticas:
- Ciencias de la computación
- Medicina y salud
- Enfermedades