Outlier Detection with Data Mining Techniques and Statistical Methods
Abstract:
The outlier detection in the field of data mining and Knowledge Discovering from Data (KDD) is capturing special interest due to its benefits. It can be applied in the financial area; because the obtained data patterns can help finding possible frauds and user errors. Therefore, it is essential to assess the truthfulness of the information. In this context, data auditory process uses techniques of data mining that play a significant role in the detection of unusual behavior. Here, a method for detecting values that can be considered as outliers in a nominal database is proposed. The basic idea in this method is to implement: a Global k-Nearest Neighbors algorithm, a clustering algorithm named k-means, and a statistical method of chi-square. The application of algorithms has been developed with a database of candidate people for the granting of a loan. Each test was made on a dataset of 1180 registers in which outliers have been introduced deliberately. The experimental results show that the method is able to detect all introduced values, which were previously labeled to be differentiated. Consequently, there were found a total of 48 tuples with outliers of 11 nominal columns.
Año de publicación:
2019
Keywords:
- -KNN
- -financial-fraud
- -chi-square
- -data-mining
- outlier
Fuente:
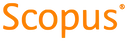
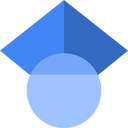
Tipo de documento:
Conference Object
Estado:
Acceso restringido
Áreas de conocimiento:
- Minería de datos
- Ciencias de la computación
Áreas temáticas:
- Ciencias de la computación