Over-sampling imbalanced datasets using the covariance matrix
Abstract:
INTRODUCTION: Nowadays, many machine learning tasks involve learning from imbalanced datasets, leading to the miss-classification of the minority class. One of the state-of-the-art approaches to "solve" this problem at the data level is Synthetic Minority Over-sampling Technique (SMOTE) which in turn uses KNearest Neighbors (KNN) algorithm to select and generate new instances. OBJECTIVES: This paper presents SMOTE-Cov, a modified SMOTE that use Covariance Matrix instead of KNN to balance datasets, with continuous attributes and binary class. METHODS: We implemented two variants SMOTE-CovI, which generates new values within the interval of each attribute and SMOTE-CovO, which allows some values to be outside the interval of the attributes. RESULTS: The results show that our approach has a similar performance as the state-of-the-art approaches. CONCLUSION: In this paper, a new algorithm is proposed to generate synthetic instances of the minority class, using the Covariance Matrix.
Año de publicación:
2020
Keywords:
- Imbalanced datasets
- Covariance matrix
- Oversampling
- Attribute dependency
Fuente:
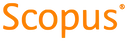
Tipo de documento:
Article
Estado:
Acceso restringido
Áreas de conocimiento:
- Estadísticas
- Optimización matemática
Áreas temáticas:
- Ciencias de la computación