A Novel Approach to Detect Missing Values Patterns in Time Series Data
Abstract:
The increase of environmental sensors to capture the behavior of cities implies large amounts of shared data. However, missing values issues are unavoidable, becoming it a critical problem for studies which require data analysis over extensive periods. The main problem is evident in longitudinal studies since they require data over long periods. Hence, a convenient process is to support the data collection rules by determining the behavior of common missing data slots. This process is possible by discovering missing data patterns over time series based on: (1) Data matrices definition, (2) Compute and categorize the missed periods using the proposed algorithm, (3) Identify the time analysis scenarios, and (4) Applying the Kernel Density Estimation algorithm. This paper describes the experimentation of this method using a real air quality dataset from Cuenca, Ecuador, collected over one-year. The results show that the proposed approach is useful to evidence the missing data patterns. Also, this approach provides a good starting point for companies and laboratories interested in improving their data collection rules.
Año de publicación:
2020
Keywords:
- Missing values patterns
- Kernel Density Estimation
- Compute missing values
Fuente:
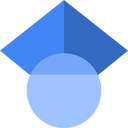
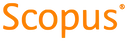
Tipo de documento:
Conference Object
Estado:
Acceso restringido
Áreas de conocimiento:
- Análisis de datos
- Ciencias de la computación
- Inferencia estadística
Áreas temáticas:
- Métodos informáticos especiales
- Programación informática, programas, datos, seguridad
- Funcionamiento de bibliotecas y archivos