A Novel Deep Neural Network that Uses Space-Time Features for Tracking and Recognizing a Moving Object
Abstract:
This work proposes a deep neural net (DNN) that accomplishes the reliable visual recognition of a chosen object captured with a webcam and moving in a 3D space. Autoencoding and substitutional reality are used to train a shallow net until it achieves zero tracking error in a discrete ambient. This trained individual is set to work in a real world closed loop system where images coming from a webcam produce displacement information for a moving region of interest (ROI) inside the own image. This loop gives rise to an emergent tracking behavior which creates a self-maintain flow of compressed space-time data. Next, short term memory elements are set to play a key role by creating new representations in terms of a space-time matrix. The obtained representations are delivery as input to a second shallow network which acts as "recognizer". A noise balanced learning method is used to fast train the recognizer with real-world images, giving rise to a simple and yet powerful robotic eye, with a slender neural processor that vigorously tracks and recognizes the chosen object. The system has been tested with real images in real time.
Año de publicación:
2017
Keywords:
- artificial vision
- deep learning
- deep architectures
Fuente:
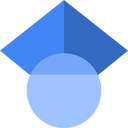
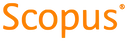
Tipo de documento:
Article
Estado:
Acceso abierto
Áreas de conocimiento:
- Red neuronal artificial
- Ciencias de la computación
- Simulación por computadora
Áreas temáticas de Dewey:
- Ciencias de la computación

Objetivos de Desarrollo Sostenible:
- ODS 9: Industria, innovación e infraestructura
- ODS 4: Educación de calidad
- ODS 8: Trabajo decente y crecimiento económico
