PURE VERSUS HYBRID TRANSFORMERS FOR MULTI-MODAL BRAIN TUMOR SEGMENTATION: A COMPARATIVE STUDY
Abstract:
Vision Transformers (ViT)-based models are witnessing an exponential growth in the medical imaging community. Among desirable properties, ViTs provide a powerful modeling of long-range pixel relationships, contrary to inherently local convolutional neural networks (CNN). These emerging models can be categorized either as hybrid-based when used in conjunction with CNN layers (CNN-ViT) or purely Transformers-based. In this work, we conduct a comparative quantitative analysis to study the differences between a range of available Transformers-based models using controlled brain tumor segmentation experiments. We also investigate to what extent such models could benefit from modality interaction schemes in a multi-modal setting. Results on the publicly-available BraTS2021 dataset show that hybrid-based pipelines generally tend to outperform simple Transformers-based models. In these experiments, no particular improvement using multi-modal interaction schemes was observed.
Año de publicación:
2022
Keywords:
- hybrid CNN-Transformers models
- tumor segmentation
- multi-modality
- vision Transformers
Fuente:
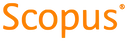
Tipo de documento:
Conference Object
Estado:
Acceso restringido
Áreas de conocimiento:
- Aprendizaje automático
- Ciencias de la computación
Áreas temáticas:
- Enfermedades