Pairing ensemble numerical weather pbkp_rediction with ensemble physical model chain for probabilistic photovoltaic power forecasting
Abstract:
Under the two-step framework of photovoltaic (PV) power forecasting, that is, forecasting first the irradiance and then converting it to PV power, there are two chief ways in which one can account for the uncertainty embedded in the final PV power forecast. One of those is to produce probabilistic irradiance forecast through, for example, ensemble numerical weather pbkp_rediction (NWP), and the other is to pass the irradiance forecast through a collection of different irradiance-to-power conversion sequences, which are known as model chains. This work investigates, for the first time, into the question: Whether pairing ensemble NWP with ensemble model chain is better than leveraging any individual method alone? Using data from 14 utility-scale ground-mounted PV plants in Hungary and the state-of-the-art global mesoscale NWP model of the European Centre for Medium-Range Weather Forecasts, it is herein demonstrated that the best probabilistic PV power forecast needs to consider both ensemble NWP and ensemble model chain. Furthermore, owing to the higher-quality probabilistic forecasts, the point forecast accuracy is also improved substantially through pairing. Overall, the recommended paring strategy achieves a mean-normalized continuous ranked probability score and a root mean square error of 18.4% and 42.1%, respectively.
Año de publicación:
2023
Keywords:
- Ensemble NWP
- Photovoltaic power
- Quantile regression
- Probabilistic solar forecasting
- ECMWF
- Physical model chains
Fuente:
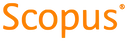
Tipo de documento:
Article
Estado:
Acceso abierto
Áreas de conocimiento:
- Meteorología
- Energía renovable
- Energía
Áreas temáticas:
- Ciencias de la computación