Pareto partial dominance MOEA and hybrid archiving strategy included CDAS in many-objective optimization
Abstract:
In this work, we propose a novel multi-objective evolutionary algorithm (MOEA) that uses Pareto partial dominance, which calculates dominance between solutions using only r objective functions selected from m objective functions to induce appropriate selection pressure in the evolution process of MOEA. Also, we temporally switch r objective functions among mCr combinations in every interval generations Ig to optimize all of the objective functions throughout the entire evolution process. In this work, we use many-objective 0/1 knapsack problems to verify the search performance of the proposed Pareto partial dominance MOEA (PPD-MOEA). Simulation results show that there is an optimum value for the number of objective functions r to be considered in Pareto partial dominance, and the interval (generation numbers) Ig to maximize the entire search performance. Also, the search performance of PPD-MOEA is superior to NSGA-II and recent state-of-the-art MOEAs, i.e., IBEA, CDAS and MSOPS. Additionally, to further enhance the search performance of PPD-MOEA, we propose a hybrid archiving strategy which uses both conventional NSGA-II and CDAS to select well-spread and well-converged solutions simultaneously when updating the archive population. Simulation results show that the hybrid archiving strategy further improves the search performance of PPD-MOEA by enhancing convergence while maintaining diversity in the archive population. © 2010 IEEE.
Año de publicación:
2010
Keywords:
Fuente:
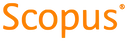
Tipo de documento:
Conference Object
Estado:
Acceso restringido
Áreas de conocimiento:
- Optimización matemática
- Optimización matemática
- Optimización matemática
Áreas temáticas de Dewey:
- Programación informática, programas, datos, seguridad
- Ciencias de la computación
- Funcionamiento de bibliotecas y archivos

Objetivos de Desarrollo Sostenible:
- ODS 9: Industria, innovación e infraestructura
- ODS 17: Alianzas para lograr los objetivos
- ODS 8: Trabajo decente y crecimiento económico
