Parkinson’s Disease Diagnosis Through Electroencephalographic Signal Processing and Sub-optimal Feature Extraction
Abstract:
Parkinson’s disease is the second most common neurological disorder after Alzheimer. Several limitations and challenges have arisen when aiming to diagnose this disease. In this regard, a computer-aided diagnosis system is enforced for the early detection of any abnormalities. Prominent research efforts have been developed based on speech and gait analysis; nonetheless, electroencephalographic (EEG)-signal-driven approaches have acquired some interest recently to diagnose an early Parkinson’s disease. According to recent studies, the angles and sharpness of brain waves may hold key hints to detect Parkinson’s disease. In the present work, an exploratory study over digital signal processing, and machine learning techniques for characterizing and classifying Parkinson-diagnosed EEG signals is conducted; waveform shape, spectral, statistical and non-linear features are taken into account for the present study. The results, without being definitive, propose a suitable set of processing techniques to increase the performance, estimation accuracy, and interpretation of this physiological phenomenon. At the end, it was found that with the characterization performed, k-NN is the classifier which performs better, obtaining a mean accuracy of 86% when differentiating Parkinson’s disease patients and healthy control subjects.
Año de publicación:
2022
Keywords:
- Waveform shape
- Parkinson’s Disease
- Machine learning
- Medical informatics
- Signal processing
- EEG
Fuente:
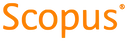
Tipo de documento:
Conference Object
Estado:
Acceso restringido
Áreas de conocimiento:
- Neurología
- Procesamiento de señales
Áreas temáticas:
- Enfermedades