Parsimonious Description of Glucose Variability in Type 2 Diabetes by Sparse Principal Component Analysis
Abstract:
Background: Abnormal glucose variability (GV) is a risk factor for diabetes complications, and tens of indices for its quantification from continuous glucose monitoring (CGM) time series have been proposed. However, the information carried by these indices is redundant, and a parsimonious description of GV can be obtained through sparse principal component analysis (SPCA). We have recently shown that a set of 10 metrics selected by SPCA is able to describe more than 60% of the variance of 25 GV indicators in type 1 diabetes (T1D). Here, we want to extend the application of SPCA to type 2 diabetes (T2D). Methods: A data set of CGM time series collected in 13 T2D subjects was considered. The 25 GV indices considered for T1D were evaluated. SPCA was used to select a subset of indices able to describe the majority of the original variance. Results: A subset of 10 indicators was selected and allowed to describe 83% of the variance of the original pool of 25 indices. Four metrics sufficient to describe 67% of the original variance turned out to be shared by the parsimonious sets of indices in T1D and T2D. Conclusions: Starting from a pool of 25 indices assessed from CGM time series in T2D subjects, reduced subsets of metrics virtually providing the same information content can be determined by SPCA. The fact that these indices also appear in the parsimonious description of GV in T1D may indicate that they could be particularly informative of GV in diabetes, regardless of the specific type of disease.
Año de publicación:
2016
Keywords:
- continuous glucose monitoring
- glucose variability
- type 1 diabetes
- Type 2 Diabetes
Fuente:
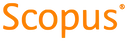
Tipo de documento:
Article
Estado:
Acceso restringido
Áreas de conocimiento:
- Diabetes
- Optimización matemática
Áreas temáticas de Dewey:
- Fisiología humana
- Enfermedades
- Salud y seguridad personal