Parsimonious models of precipitation phase derived from random forest knowledge: Intercomparing logistic models, neural networks, and random forest models
Abstract:
The precipitation phase (PP) affects the hydrologic cycle which in turn affects the climate system. A lower ratio of snow to rain due to climate change affects timing and duration of the stream flow. Thus, more knowledge about the PP occurrence and drivers is necessary and especially important in cities dependent on water coming from glaciers, such as Quito, the capital of Ecuador (2.5 million inhabitants), depending in part on the Antisana glacier. The logistic models (LM) of PP rely only on air temperature and relative humidity to pbkp_redict PP. However, the processes related to PP are far more complex. The aims of this study were threefold: (i) to compare the performance of random forest (RF) and artificial neural networks (ANN) to derive PP in relation to LM; (ii) to identify the main drivers of PP occurrence using RF; and (iii) to develop LM using meteorological drivers derived from RF. The results show that RF and ANN outperformed LM in pbkp_redicting PP in 8 out of 10 metrics. RF indicated that temperature, dew point temperature, and specific humidity are more important than wind or radiation for PP occurrence. With these pbkp_redictors, parsimonious and efficient models were developed showing that data mining may help in understanding complex processes and complements expert knowledge.
Año de publicación:
2021
Keywords:
- Andes precipitation
- Precipitation phase
- Logistic models
- Automatic discovery
- random forest
Fuente:
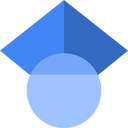
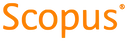
Tipo de documento:
Article
Estado:
Acceso abierto
Áreas de conocimiento:
- Aprendizaje automático
- Inferencia estadística
Áreas temáticas:
- Programación informática, programas, datos, seguridad
- Métodos informáticos especiales
- Ingeniería y operaciones afines