Particle Swarm Clustering in clustering ensembles: Exploiting pruning and alignment free consensus
Abstract:
A clustering ensemble combines in a consensus function the partitions generated by a set of independent base clusterers. In this study both the employment of particle swarm clustering (PSC) and ensemble pruning (i.e., selective reduction of base partitions) using evolutionary techniques in the design of the consensus function is investigated. In the proposed ensemble, PSC plays two roles. First, it is used as a base clusterer. Second, it is employed in the consensus function; arguably the most challenging element of the ensemble. The proposed consensus function exploits a representation for the base partitions that makes cluster alignment unnecessary, allows for the combination of partitions with different number of clusters, and supports both disjoint and overlapping (fuzzy, probabilistic, and possibilistic) partitions. Results on both synthetic and real-world data sets show that the proposed ensemble can produce statistically significant better partitions, in terms of the validity indices used, than the best base partition available in the ensemble. In general, a small number of selected base partitions (below 20% of the total) yields the best results. Moreover, results produced by the proposed ensemble compare favorably to those of state-of-the-art clustering algorithms, and specially to swarm based clustering ensemble algorithms.
Año de publicación:
2017
Keywords:
- ensembles
- Consensus function
- Overlapping partitions
- Particle Swarm Optimization
- Particle swarm clustering
- Clustering
Fuente:
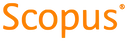
Tipo de documento:
Article
Estado:
Acceso restringido
Áreas de conocimiento:
- Minería de datos
- Ciencias de la computación
- Ciencias de la computación
Áreas temáticas:
- Ciencias de la computación