A Novel Neural-Fuzzy Method to Search the Optimal Step Size for NLMS Beamforming
Abstract:
This paper presents a novel algorithm based on neural networks and fuzzy logic to generate membership functions and search an approximation of the optimal step-size for Normalized Least Mean Squares (NLMS) beamforming systems. The proposed method makes a new error curve, Error Ensemble Learning (EEL), based on the final estimated value of the adaptive algorithm's mean-square-error. A fuzzy clustering method individually assigns membership values to each EEL curve coordinates. This information is fed into a neural network to generate membership functions for a fuzzy inference system. The final estimation of the optimal step-size is obtained using a group of Mamdani linguistic propositions and the centroid defuzzification method. Simulation results show that a useful approximation of the optimal step-size is obtained for different interference conditions; the evaluation results also show that a higher directivity is achieved in the radiation beam pattern.
Año de publicación:
2015
Keywords:
- BEAMFORMING
- NLMS algorithm
- Neural networks
- fuzzy logic
- Adaptive filters
Fuente:
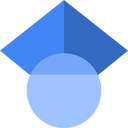
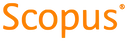
Tipo de documento:
Article
Estado:
Acceso restringido
Áreas de conocimiento:
- Algoritmo
- Aprendizaje automático
- Control óptimo
Áreas temáticas:
- Métodos informáticos especiales
- Economía
- Física aplicada