Pattern recognition of soldier uniforms with dilated convolutions and a modified encoder-decoder neural network architecture
Abstract:
In this paper, we study a deep learning (DL)-based multimodal technology for military, surveillance, and defense applications based on a pixel-by-pixel classification of soldier’s image dataset. We explore the acquisition of images from a remote tactical-robot to a ground station, where the detection and tracking of soldiers can help the operator to take actions or automate the tactical-robot in battlefield. The soldier detection is achieved by training a convolutional neural network to learn the patterns of the soldier’s uniforms. Our CNN learns from the initial dataset and from the actions taken by the operator, as opposed to the old-fashioned and hard-coded image processing algorithms. Our system attains an accuracy of over 81% in distinguishing the specific soldier uniform and the background. These experimental results prove our hypothesis that dilated convolutions can increase the segmentation performance when compared with patch-based, and fully connected networks.
Año de publicación:
2021
Keywords:
Fuente:
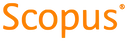
Tipo de documento:
Article
Estado:
Acceso abierto
Áreas de conocimiento:
- Red neuronal artificial
- Ciencias de la computación
Áreas temáticas:
- Ciencias de la computación