People Identification through Facial Recognition using Deep Learning
Abstract:
Nowadays, Closed Circuit Television (CCTV) monitoring systems, access control, and many other security-related applications incorporate facial recognition techniques. This disruptive tool differs from other biometric techniques, as faces can be recognized remotely. Thus, these applications can be incorporated in different institutions with the purpose of restricting access to unauthorized people, avoiding damages and losses to the property. The objective of this study is to identify people in controlled and uncontrolled environments within a university building that has suffered insecurity problems on several occasions. For this reason, it is used a convolutional neural network (CNN) architecture that was implemented on a GPU. The procedure consists of 3 phases: (i) training; (ii) learning and; (iii) classification. The CNN training stage was performed using the VGGFace2 dataset. The learning and generalization of deeply discriminatory facial embeddings of 512 bytes per face by joint supervision of softmax loss and center loss signals. The classification used a support vector machine (SVM) in several experiments with different amounts of classes. The efficiency of this approach was tested in real time on a sample of 128 students by means of quantitative metrics derived by confusion matrix. The overall accuracy in controlled and uncontrolled contexts was 96% and 71.43%, respectively. The proposed method establishes a starting point for the development of a more robust system in production environments.
Año de publicación:
2019
Keywords:
- SVM
- Facial recognition
- cnn
- deep learning
- Machine learning
Fuente:
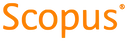
Tipo de documento:
Conference Object
Estado:
Acceso restringido
Áreas de conocimiento:
- Inteligencia artificial
- Ciencias de la computación
- Ciencias de la computación
Áreas temáticas:
- Métodos informáticos especiales
- Percepción, movimiento, emociones y pulsiones
- Física aplicada