Performance comparison in network traffic pbkp_rediction for polynomial regression to P1P versus ARIMA and MWM
Abstract:
This document presents a performance analysis on network data traffic channel mathematical modeling using polynomial regression applied to Potential Polynomials of degree one (P1P) to propose an easier computational alternative for traditional methods and even those whose uses neural networks. It proposes an alternative to traditional methods that use neural networks. There are several studies that look into modeling and traffic pbkp_rediction as auto-regression in movement average (ARIMA) or Wavelet based. In this sense, this paper analyzes the Mean Absolute Error (MAE) and the Mean Square Error (MSE) to compare P1P polynomial regression other mentioned methods. Proofs were realized with a set of 100 sample signals from different network traffics analyzing TCP and UDP packets to compute average MAE and MSE values. Finally, graphics will demonstrate the statistical performance of the proposed method, and comparative tables with different modeling and pbkp_redictor algorithms will be presented.
Año de publicación:
2018
Keywords:
- ARIMA
- P1P
- Traffic Pbkp_rediction
- MWM
Fuente:
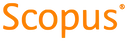
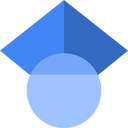
Tipo de documento:
Conference Object
Estado:
Acceso restringido
Áreas de conocimiento:
- Análisis de datos
- Ciencias de la computación
- Estadísticas
Áreas temáticas:
- Ciencias de la computación