A Recommender System for Improving Median Plane Sound Localization Performance Based on a Nonlinear Representation of HRTFs
Abstract:
We propose a new method to improve median plane sound localization performance using a nonlinear representation of head-related transfer functions (HRTFs) and a recommender system. First, we reduce the dimensionality of an HRTF data set with multiple subjects using manifold learning in conjunction with a customized intersubject graph which takes into account relevant prior knowledge of HRTFs. Then, we use a sound localization model to estimate a subject's localization performance in terms of polar error and quadrant error rate. These metrics are merged to form a single rating per HRTF pair that we feed into a recommender system. Finally, the recommender system takes the low-dimensional HRTF representation as well as the ratings obtained from the localization model to pbkp_redict the best HRTF set, possibly constructed by mixing HRTFs from different individuals, that minimizes a subject's localization error. The simulation results show that our method is capable of choosing a set of HRTFs that improves the median plane localization performance with respect to the mean localization performance using non-individualized HRTFs. Moreover, the localization performance achieved by our HRTF recommender system shows no significant difference to the localization performance observed with the best matching non-individualized HRTFs but with the advantage of not having to perform listening tests with all individuals' HRTFs from the database.
Año de publicación:
2018
Keywords:
- HRTF
- Manifold learning
- spatial audio
- recommender systems
Fuente:
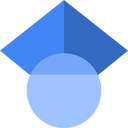
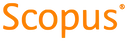
Tipo de documento:
Article
Estado:
Acceso abierto
Áreas de conocimiento:
- Ciencias de la computación
- Aprendizaje automático
Áreas temáticas:
- Física aplicada
- Métodos informáticos especiales