Plant Disease Classification and Severity Estimation: A Comparative Study of Multitask Convolutional Neural Networks and First Order Optimizers
Abstract:
The detection of plant diseases has been a hot research topic lately, specially since deep learning models and state-of-the-art convolutional neural networks (CNNs) architectures came into play. For this reason, this paper aims to compare several multitask CNN architectures used for: (i) classifying the environmental stress of coffee leaves, and (ii) estimating the severity of diseases that affect coffee plantations. This study is performed in two stages. First, the best performing CNN architecture was obtained from the multitask tests, which was ResNet34. Second, we improved the performance of ResNet34 by training it with six different optimization functions, and three different initial learning rates. This comparison was based on the analysis of different performance metrics such as the classification accuracy, training loss, F1-score, and the required number of epochs to achieve convergence. Our results show that with an initial learning rate of 1 × 10 - 3, Adagrad and Adam are the best optimizers for disease classification and severity estimation, respectively. Likewise, stochastic gradient descent shows to be an acceptable optimizer when the momentum hyperparameter is tuned properly.
Año de publicación:
2022
Keywords:
- Artificial Intelligence
- Optimizers
- Severity estimation
- Agriculture technology
- Disease classification
- neural network
- Computer Vision
Fuente:
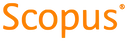
Tipo de documento:
Conference Object
Estado:
Acceso restringido
Áreas de conocimiento:
- Fitopatología
- Aprendizaje automático
- Ciencias de la computación
Áreas temáticas:
- Agricultura y tecnologías afines
- Métodos informáticos especiales
- Programación informática, programas, datos, seguridad