A Review on Neurocomputing Based wind Turbines Fault Diagnosis and Prognosis
Abstract:
Renewable energy, especially wind power energy, has gone through fast growth in the last decade According to the Global Wind Energy Council (GWEC), the worldwide global installed wind power capacity has reached 486.8 GW in 2016 and may reach 817 GW by 2021. Commonly, wind turbines (WTs) are located in remote areas with limited access and their maintenance and repairing operations often require the use of expensive equipment such as cranes and lifting machinery, increasing significantly the operation and maintenance (O&M) costs that may far exceed the cost of the failed component itself. Moreover, one of the main sources for the O&M costs is the unscheduled maintenance due to unexpected failures. Therefore, fault diagnosis and prognosis are critical to help reducing O&M costs and keeping wind power a competitive energy source. The main contribution of this paper is an updated, unbiased and repeatable search, review and analysis (summary and classification) of the main approaches, e.g. methods and techniques, for WTs fault diagnosis and prognosis. A systematic review of neurocomputing based approaches is presented, including innovative comprehensive lists of references grouped by the studied WT components. The paper concludes with a discussion on some promising future research directions.
Año de publicación:
2018
Keywords:
- Artificial Neural
- Fault prognosis
- Data-driven approaches
- Wind turbine
- Fault diagnosis
- deep learning
- Machine learning
Fuente:
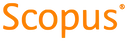
Tipo de documento:
Conference Object
Estado:
Acceso restringido
Áreas de conocimiento:
- Energía renovable
- Inteligencia artificial
Áreas temáticas:
- Métodos informáticos especiales