Polynomial Chaos based on the parallelized ensemble Kalman filter to estimate precipitation states
Abstract:
This article develops a methodology combining methods of numerical analysis and stochastic differential equations with computational algorithms to treat problems which have complex nonlinear dynamics in high dimensions. A method to estimate parameters and states of a dynamic system is proposed inspired by the parallelized ensemble Kalman filter (PEnKF) and the polynomial chaos theory of Wiener-Askey. The main advantage of the proposal is in providing a precise efficient algorithm with low computational cost. For the analysed data, the methods provide good predictions, spatially and temporally, for the unknown precipitation states for the first 24 hours. Two goodness of fit measures provide confidence in the quality of the model predictions. The performance of the parallel algorithm, measured by the acceleration and efficiency factors, shows an increase of 7% in speed with respect to the sequential version and is most efficient for P = 2 threads.
Año de publicación:
2015
Keywords:
- Stochastic differential equations
- Parallelized ensemble Kalman filter
- Numerical Methods
- Polynomial chaos
Fuente:
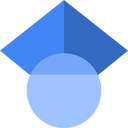
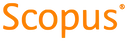
Tipo de documento:
Article
Estado:
Acceso abierto
Áreas de conocimiento:
- Análisis numérico
- Optimización matemática
- Hidrología
Áreas temáticas de Dewey:
- Ciencias de la computación

Objetivos de Desarrollo Sostenible:
- ODS 9: Industria, innovación e infraestructura
- ODS 13: Acción por el clima
- ODS 17: Alianzas para lograr los objetivos
